The Number Of Principal Components
mmpmp
If the last paragraph’s line of reasoning seems to contain a gap, it is in the failure todistinguish between sampling error and measurement error. Significance tests concern onlysampling error, but it is reasonable to hypothesize that an observed correlation of, say, .8differsfrom 1.0 only because of measurement error. However, the possibility of measurement errorimplies that you should be thinking in terms of a common factor model rather a componentmodel, since measurement error implies that there is some variance in each X-variable notexplained by the factors.
Types Of Factor Extraction
Principal component analysis is a widely used method for factor extraction, which is the first phase of EFA. Factor weights are computed to extract the maximum possible variance, with successive factoring continuing until there is no further meaningful variance left. The factor model must then be rotated for analysis.
Canonical factor analysis, also called Rao’s canonical factoring, is a different method of computing the same model as PCA, which uses the principal axis method. Canonical factor analysis seeks factors which have the highest canonical correlation with the observed variables. Canonical factor analysis is unaffected by arbitrary rescaling of the data.
Common factor analysis, also called principal factor analysis or principal axis factoring , seeks the fewest factors which can account for the common variance of a set of variables.
Image factoring is based on the correlation matrix of predicted variables rather than actual variables, where each variable is predicted from the others using multiple regression.
Alpha factoring is based on maximizing the reliability of factors, assuming variables are randomly sampled from a universe of variables. All other methods assume cases to be sampled and variables fixed.
Factor regression model is a combinatorial model of factor model and regression model or alternatively, it can be viewed as the hybrid factor model, whose factors are partially known.
Higher Order Factor Analysis
This article may be confusing or unclear to readers. Please help clarify the article. There might be a discussion about this on the talk page. |
Higher-order factor analysis is a statistical method consisting of repeating steps factor analysis â oblique rotation â factor analysis of rotated factors. Its merit is to enable the researcher to see the hierarchical structure of studied phenomena. To interpret the results, one proceeds either by post-multiplying the primary factor pattern matrix by the higher-order factor pattern matrices and perhaps applying a Varimax rotation to the result or by using a Schmid-Leiman solution which attributes the variation from the primary factors to the second-order factors.
Don’t Miss: Ccl4 Molecular Geometry
Properties Of The Data
As with any statistical analysis, before performing a factor analysis the researcher must investigate whether the data meet the assumptions for the proposed analysis. Section 1 of the Supplemental Material provides a summary of what a researcher should check for in the data for the purposes of meeting the assumptions of a factor analysis and an illustration applied to the example data. These include analyses of missing values, outliers, factorability, normality, linearity, and multicollinearity. Box 3 provides an example of how to report these analyses in a manuscript.
In Physical And Biological Sciences
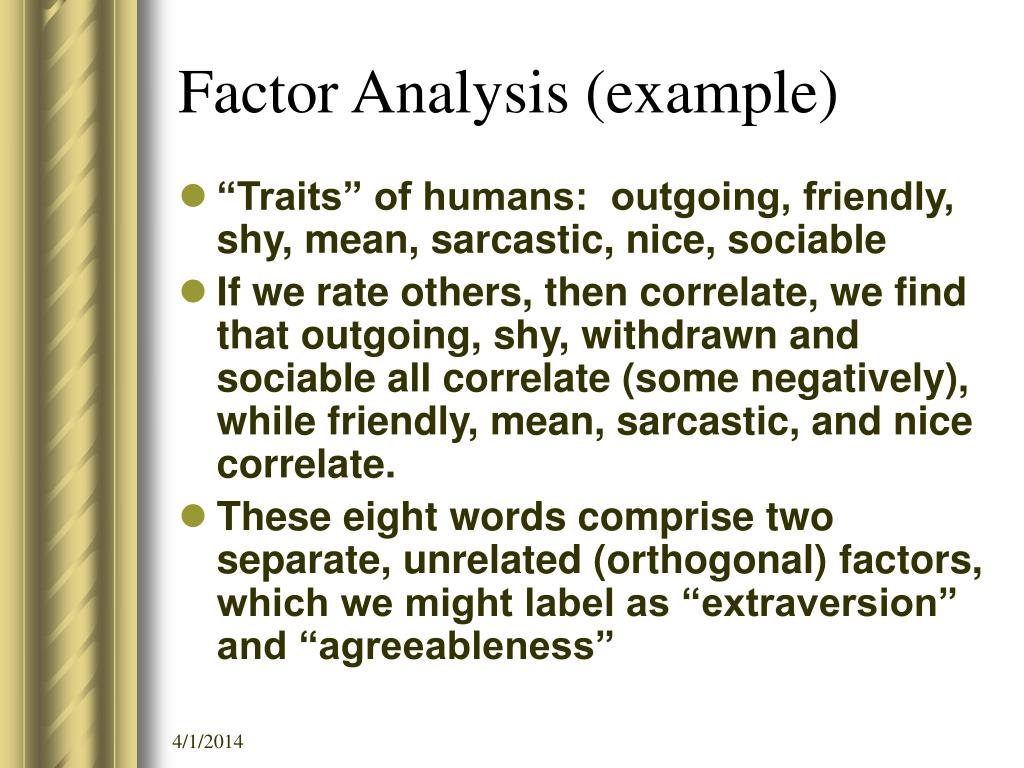
Factor analysis has also been widely used in physical sciences such as geochemistry, hydrochemistry,astrophysics and cosmology, as well as biological sciences, such as ecology, molecular biology, neuroscience and biochemistry.
In groundwater quality management, it is important to relate the spatial distribution of different chemicalparameters to different possible sources, which have different chemical signatures. For example, a sulfide mine is likely to be associated with high levels of acidity, dissolved sulfates and transition metals. These signatures can be identified as factors through R-mode factor analysis, and the location of possible sources can be suggested by contouring the factor scores.
In geochemistry, different factors can correspond to different mineral associations, and thus to mineralisation.
Read Also: Which Founding Contributors To Psychology Helped Develop Behaviorism
Key Concepts In Factor Analysis
One of the most important ideas in factor analysis is variance how much your numerical values differ from the average. When you perform factor analysis, youre looking to understand how the different underlying factors influence the variance among your variables. Every factor will have an influence, but some will explain more variance than others, meaning that the factor more accurately represents the variables its comprised of.
The amount of variance a factor explains is expressed in an eigenvalue. If a factor solution has an eigenvalue of 1 or above, it explains more variance than a single observed variable which means it can be useful to you in cutting down your number of variables. Factor solutions with eigenvalues less than 1 account for less variability than a single variable and are not retained in the analysis. In this sense, a solution would contain fewer factors than the original number of variables.
Another important metric is factor score. This is a numerical measure that describes how strongly a variable from the original research data is related to a given factor. Another term for this association or weighting towards a certain factor is factor loading.
Factor Analysis Versus Clustering And Multidimensional Scaling
Another advantage of factor analysis over these othermethods is that factor analysis can recognize certain properties ofcorrelations. For instance, if variables A and B each correlate .7with variable C, and correlate .49 with each other, factor analysiscan recognize that A and B correlate zero when C is held constantbecause .72 = .49. Multidimensional scaling andcluster analysis have no ability to recognize such relationships,since the correlations are treated merely as generic “similaritymeasures” rather than as correlations.
We are not saying these other methods should never beapplied to correlation matrices sometimes they yield insights notavailable through factor analysis. But they have definitely notmade factor analysis obsolete. The next section touches on thispoint.
Also Check: Exponential Growth And Decay Common Core Algebra 1 Homework Answers
How Are Pca Loadings Calculated
Loadings are interpreted as the coefficients of the linear combination of the initial variables from which the principal components are constructed. From a numerical point of view, the loadings are equal to the coordinates of the variables divided by the square root of the eigenvalue associated with the component.
Criteria For Determining The Number Of Factors
Researchers wish to avoid such subjective or arbitrary criteria for factor retention as “it made sense to me”. A number of objective methods have been developed to solve this problem, allowing users to determine an appropriate range of solutions to investigate. Methods may not agree. For instance, the parallel analysis may suggest 5 factors while Velicer’s MAP suggests 6, so the researcher may request both 5 and 6-factor solutions and discuss each in terms of their relation to external data and theory.
Modern criteria
Horn’s parallel analysis : A Monte-Carlo based simulation method that compares the observed eigenvalues with those obtained from uncorrelated normal variables. A factor or component is retained if the associated eigenvalue is bigger than the 95th percentile of the distribution of eigenvalues derived from the random data. PA is among the more commonly recommended rules for determining the number of components to retain, but many programs fail to include this option ” rel=”nofollow”> R). However, Formann provided both theoretical and empirical evidence that its application might not be appropriate in many cases since its performance is considerably influenced by sample size, item discrimination, and type of correlation coefficient.
Older methods
Variance explained criteria: Some researchers simply use the rule of keeping enough factors to account for 90% of the variation. Where the researcher’s goal emphasizes parsimony , the criterion could be as low as 50%.
Also Check: Who Are Paris Jackson’s Biological Parents
Pros And Cons Of Factor Analysis
Factor analysis explores large dataset and finds interlinked associations. It reduces the observed variables into a few unobserved variables or identifies the groups of inter-related variables, which help the market researchers to compress the market situations and find the hidden relationship among consumer taste, preference, and cultural influence. Also, It helps in improve questionnaire in for future surveys. Factors make for more natural data interpretation.
Results of factor analysis are controversial. Its interpretations can be debatable because more than one interpretation can be made of the same data factors. After factor identification and naming of factors requires domain knowledge.
Differences In Procedure And Results
The differences between PCA and factor analysis are further illustrated by Suhr :
- PCA results in principal components that account for a maximal amount of variance for observed variables FA accounts for common variance in the data.
- PCA inserts ones on the diagonals of the correlation matrix FA adjusts the diagonals of the correlation matrix with the unique factors.
- PCA minimizes the sum of squared perpendicular distance to the component axis FA estimates factors which influence responses on observed variables.
- The component scores in PCA represent a linear combination of the observed variables weighted by eigenvectors the observed variables in FA are linear combinations of the underlying and unique factors.
- In PCA, the components yielded are uninterpretable, i.e. they do not represent underlying âconstructsâ in FA, the underlying constructs can be labelled and readily interpreted, given an accurate model specification.
The basic steps are:
- Identify the salient attributes consumers use to evaluate products in this category.
- Use quantitative marketing research techniques to collect data from a sample of potential customers concerning their ratings of all the product attributes.
- Input the data into a statistical program and run the factor analysis procedure. The computer will yield a set of underlying attributes .
Also Check: Ct Algebra 1 Curriculum Version 3.0 Activity 4.5 5 Answers
Arguments Contrasting Pca And Efa
Fabrigar et al. address a number of reasons used to suggest that PCA is not equivalent to factor analysis:
The Use Of Surveys In Biology Education Research
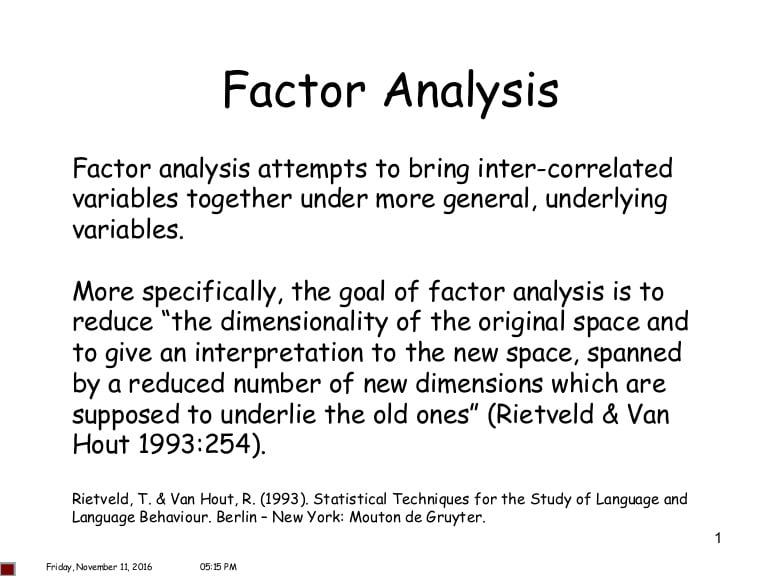
Surveys and achievement tests are common tools used in biology education research to measure students attitudes, feelings, and knowledge. In the early days of biology education research, researchers designed their own surveys to obtain information about students. Generally, each question on these instruments asked about something different and did not involve extensive use of measures of validity to ensure that researchers were, in fact, measuring what they intended to measure . In recent years, researchers have begun adopting existing measurement instruments. This shift may be due to researchers increased recognition of the amount of work that is necessary to create and validate survey instruments . While this shift is a methodological advancement, as a community of researchers we still have room to grow. As biology education researchers who use surveys, we need to understand both the theoretical and statistical underpinnings of validity to appropriately employ instruments within our contexts. As a community, biology education researchers need to move beyond simply adopting a validated instrument to establishing the validity of the scores produced by the instrument for a researchers intended interpretation and use. This will allow education researchers to produce more rigorous and replicable science. In this primer, we walk the reader through important validity aspects to consider and report when using surveys in their specific context.
Also Check: Who Are Paris Jackson’s Biological Parents
Factor Analysis: Evidence Of Dimensionality Among A Set Of Items
Factor analysis is a statistical technique that analyzes the relationships between a set of survey items to determine whether the participants responses on different subsets of items relate more closely to one another than to other subsets, that is, it is an analysis of the dimensionality among the items . This technique was explicitly developed to better elucidate the dimensionality underpinning sets of achievement test items . Speaking in terms of constructs, factor analysis can be used to analyze whether it is likely that a certain set of items together measure a predefined construct . Factor analysis can broadly be divided into exploratory factor analysis and confirmatory factor analysis .
Types Of Factor Analysis
Exploratory factor analysis
Exploratory factor analysis is used to identify complex interrelationships among items and group items that are part of unified concepts. The researcher makes no a priori assumptions about relationships among factors.
Confirmatory factor analysis
Confirmatory factor analysis is a more complex approach that tests the hypothesis that the items are associated with specific factors. CFA uses structural equation modeling to test a measurement model whereby loading on the factors allows for evaluation of relationships between observed variables and unobserved variables. Structural equation modeling approaches can accommodate measurement error, and are less restrictive than least-squares estimation. Hypothesized models are tested against actual data, and the analysis would demonstrate loadings of observed variables on the latent variables , as well as the correlation between the latent variables.
Don’t Miss: Eoc Fsa Warm Ups Algebra 1 Answers
Advantages And Disadvantages Of Cost Benefit Analysis
The treatment of intangibles and the problem of equity have been discussedabove. These issues represent limitations of the method in the sense that neither is addressedautomatically in the cost-benefit process. If the decision-maker is to be in a position properlyto take account of intangible considerations and equity concerns, the analyst must, in a sense,go beyond the ordinary requirements of a cost-benefit analysis. Similarly, when the decision-makers interest is naturally focused on the bottom line, it iseasy for the analysis itself to be rather obscure. No analysis is better than the assumptions onwhich it is based and, in the interest of quality control, assumptions should always be madeexplicit.
Interpreting The Outputs From Cfa
After making all the suggested analytical decisions, a researcher is now ready to apply a CFA to the data. Model fit indices that the researcher a priori decided to use are the first element of the output that should be interpreted from a CFA. If these indices suggest that the data do not fit the specified model, then the researcher does not have empirical support for using the hypothesized survey structure. This is exactly what happened when we initially ran a CFA on Diekmans goal-endorsement instrument example . In this case, focus should shift to understanding the source of the model misfit. For example, one should ask whether there are any items that do not seem to correlate with their specified latent factor, whether any correlations seem to be missing, or whether some items on a factor group together more strongly than other items on that same factor. These questions can be answered by analyzing factor loadings, correlation residuals, and modification indices. In the following sections, we describe these in more detail. See Boxes 3, 6, and 7 for examples of how to discuss and present output from a CFA in a paper.
BOX 3. How to interpret and report CFA output for publication using the goal-endorsement example, initial CFA
Descriptive statistics
Interpreting output from the initial two-factor CFA
Factor Loadings.
Correlation Residuals.
Modification Indices.
When the Model Fit Is Good.
Also Check: Geometry Dash Practice Mode
Algorithmic Trading Research Paper
To test the whole model, we should obtain the percentages of accurate predictions for different network topologies, different transfer functions, and different combinations of these basic technical indicators. Finally, the investment return achieved from the proposed trading system should be compared with the results if the buy-and-hold strategy is
Matrix Decomposition And Rank
optional
The central theorem of factor analysis is that you can do something similar for anentire covariance matrix. A covariance matrix R can be partitioned into a common portion Cwhich is explained by a set of factors, and a unique portion U unexplained by those factors. In matrix terminology, R = C + U, which means that each entry in matrix R is the sum ofthe corresponding entries in matrices C and U.
As in analysis of variance with equal cell frequencies, the explained component C canbe broken down further. C can be decomposed into component matrices c1,c2, etc., explained by individual factors. Each of these one-factorcomponents cj equals the “outer product” of a column of “factor loadings”. The outer product of a column of numbers is the square matrix formed by letting entryjk in the matrix equal the product of entries j and k in thecolumn. Thus if a column has entries .9, .8, .7, .6, .5, as in the earlier example, its outerproduct is
.81 .72 .63 .54 .45 .72 .64 .56 .48 .40c1 .63 .56 .49 .42 .35 .54 .48 .42 .36 .30 .45 .40 .35 .30 .25
g
In the example there is only one common factor, so matrix C for this example is C55 = c1. Therefore the residual matrix U for thisexample is U55 = R55 – c1. This gives the followingmatrixfor U55:
.19 .00 .00 .00 .00 .00 .36 .00 .00 .00U55 .00 .00 .51 .00 .00 .00 .00 .00 .64 .00 .00 .00 .00 .00 .75
Don’t Miss: Eoc Fsa Warm Ups Algebra 1 Answers