Effect Of Reaction Conditions
There are no major differences in performance when considering reactions at different conditions. Figure 14 shows the Within-n results for all the reactions conditions for differenct values of n over all cross-validation experiments. The reaction conditions with the largest number of queries show greater than 90% Within-0 values. However, all conditions show similar performance on the close rankings. When n 2, then all reaction conditions exhibit > 95% recovery perfomance.
Within-n predicted reaction recovery for different reaction conditions over cross-validation experiments. The fraction of reactant systems in which all productive reactions are recovered is presented on the y-axis, and the n is presented on the x-axis. Color and symbols are used to denoted different reaction conditions. The number of queries with the given reaction conditions are presented in parentheses after the conditions name. Details of the reaction conditions and how they map back to the Reaction Explorer reagent models are presented in Table S1.
How To Predict Products In Chemical Reactions
Chemistry students typically experience difficulty in predicting the products of chemical reactions. With practice, however, the process becomes progressively easier.
The first step—identifying the type of reaction involved—is usually the most difficult. The primary reaction types students encounter are displacement, acid-base and combustion. They are easily identified if the tell-tale signs are known. Displacement reactions involve two ionic compounds with cations and anions, such as sodium sulfate, in which sodium is the cation and sulfate is the anion. Ionic compounds always consist of a metal and a nonmetal or polyatomic anion. Decomposition reactions involve a single compound breaking into two or more compounds. Acid-base reactions must involve an acid . Combustion reactions involve hydrogen or a hydrocarbon reacting with oxygen .
What Is Chemical Reaction
A chemical reaction is a process in which one or more reactants are converted into one or more products. The substance may be either chemical compounds or chemical elements. In this process, the constituent atoms of the reactants rearrange to produce different products. For example, when iron and oxygen combine together, it produces rust.
Recommended Reading: How To Think Straight About Psychology
Ranking Provides Flexible Results
By identifying the productive reactions through ranking, our framework provides flexible and interpretable results. To assess this, we look at the predictions of the ranking system on reactants and conditions in the testing sets of cross-validation splits, i.e., the reactions shown are not seen during training. For example, two ring-forming systems over which productive reactions are correctly ranked are shown in Figure 10. These are the productive reactions for the given reactants under the Reaction Explorer Mix Reactants, Polar Protic reagent model. In cross-validation experiments, these are always the top ranked reactions with the reaction conditions corresponding to the reagent model. For the 5-member ring forming reaction in Figure 10, the reaction proceeds with the oxygen acting as a nucleophile, and the end product is a heterocycle . However another reasonable, though not as favorable, reaction could occur with an enolate nucleophile and a cyclopentanone product, though this reaction is not labeled as productive by Reaction Explorer. The ranking method correctly returns this particular reaction as the second highest ranked for this set of reactants and conditions.
How Do I Predict Products Given Only The Formulas Of The Reactants
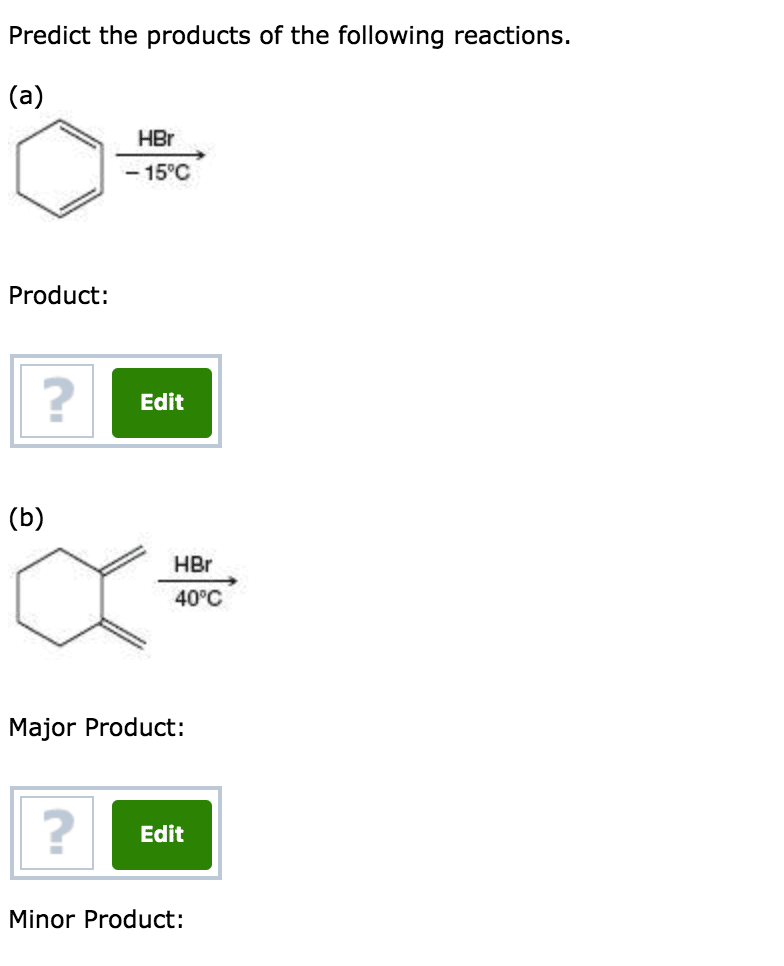
- Balance this equation, please.
-
This reaction involves two soluble ionic compoundsand so is a potential double-displacement reaction. Follow this procedure to write balancedmolecular equations for double displacement reactions, when you know only the formulas of the reactants:
You May Like: What Is Conformation In Chemistry
Artificial Neural Network Training
Before training, all features are normalized to using the minimum and maximum values of the training set. Then because the tuples labeled reactive comprise less than 10% of the data for either filled or unfilled, we oversample sites labeled reactive to ensure approximately balanced classes.
We train artificial neural networks using sigmoidal activation functions in a single hidden layer and a single output node. After some experimentation, an architecture of 10 hidden nodes was chosen. Gradients on the weights of the neural network are calculated with the standard back-propagation algorithm and a L2 regularized cross-entropy error function. The weights are optimized by stochastic gradient descent with per weight adaptive learning rates.48 Optimization is stopped after 100 epochs as this is observed to be sufficient for convergence. The end result of training is a neural network model which given an input feature vector outputs a probability of the tuple being labeled reactive.
Investigation Of Specific Reaction Classes
We investigate in detail three reaction classes that are commonly used in medicinal chemistry. Through these examples, we illustrate each of the three branches in Fig. a. We first examine the selective epoxidation of alkenes which is an example where the Molecular Transformer is producing the right prediction for the right reason. We then turn to the DielsAlder reaction, which is a scaffold-building transformation widely used in synthesis. We show that the Molecular Transformer is not able to predict this reaction. Following the bottom branch of Fig. a, we investigate it using data attribution and find that the USPTO dataset contains very few instances of DielsAlder reactions, likely explaining why the model is not able to predict the outcome correctly.
Finally, we consider the FriedelCrafts acylation reactions of substituted benzenes. We show that the Molecular Transformer predicts the right product for the wrong reason and validate our interpretation using a number of adversarial examples. We also demonstrate with the help of an artificial dataset how this behaviour is the result of dataset bias.
Epoxidation
Epoxidation reactions can be regioselective, with more substituted alkenes reacting faster because they are more electron-rich. A typical example reaction showing this type of selectivity is shown in Fig. a.
Fig. 2: IG attributions highlighting correct reasoning.
DielsAlder
Fig. 3: Data attribution explains erroneous prediction.
FriedelCrafts acylation
Also Check: The Sketch Has No Contour Geometry Solidworks
Is Organic Chem Hard
Organic chemistry is one of the hardest science subjects. Its failure and retake rates are high, and its class grade average is low. Itâs also very time-consuming, difficult to apply, and heavy on theoretical detail. If you havenât done a general chemistry course first, you could really struggle.
Machine Learning Stage : Reactive Site Filtering
Recall that we wish to train two separate classifiers to predict the filled and unfilled reactivity labels of an atom. The feature descriptions and machine learning implementations used are exactly the same for the two separate problems, except for the different labels. As such, a general reactivity labeled dataset will be considered as instances of , i.e. an atom a, a set of conditions c, and a label l , where l = 1, if is labeled reactive, and l = 0 otherwise.
You May Like: How Did Northern European Geography And Technology Affect Each Other
Ai Translates Chemistry To Predict Reaction Outcomes
Machine learning tool predicts products of organic reactions by treating chemistry like language
IBM researchers have developed a program that can predict the products of organic chemistry reactions.1 Modelled on the latest language translation systems like Googles artificial neural network the AI picked the right product 80% of the time despite not having been taught any organic chemistry rules.
What this tool is trying to do is imitate a top pro chemist in more or less the entire domain of organic chemistry, says Teodoro Laino, one of the researchers involved in the study at IBM in Zurich, Switzerland. His ambitious goal is shared by other chemists who have been attempting to create a functioning AI chemist since the 1970s, when organic chemist E J Corey kick-started the field by creating a chemical knowledge database.
However, making a tool based on chemistry knowledge can be time-consuming Bartosz Grzybowskis team took 10 years to encode their Chematica retrosynthesis program with 20,000 chemical rules. Moreover, a knowledge-based AI has difficulty tackling reactions that lie outside of its rule set. Theres a way to learn organic chemistry thats not memorising chemical rules, by just trying to find out the underlying patterns in reactions and trying to rationalise them, Laino says, explaining the approach that his team took.
Found in translation: the program can correctly predict the outcomes of reactions in four out of five instances
Models And Experimental Pipeline
We base our models directly on the reaction fingerprint models by Schwaller et al . We use a fixed-size encoder model size, tuning only the hyperparameter for dropout rate and learning rate, thus avoiding often-encountered difficulties of neural networks with numerous hyperparameters. During our experiments, we observed good performances for a wide range of dropout rates and conclude that the initial learning rate is the most important hyperparameter to tune. Figures S26S30 show hyperparameter optimisation plots . To facilitate the training, our work uses simpletransformers , a huggingface transformer and the PyTorch framework . The overall pipeline is shown in figure 1.
To provide an input compatible with the rxnfp model we use the same RDKit reaction canonicalisation and SMILES tokenization as in the rxnfp work .
Read Also: Bju Algebra 1 3rd Edition
Design Your Retrosynthesis Using Either The Automatic Or The Interactive Mode
The Retrosynthetic Pathways predictor accounts for all the relevant aspects examined by humans when designing a Retrosynthesis. Our models for Chemical Reaction and Retrosynthesis prediction are trained on a set of 2.5 million Chemical Reactions.In automatic mode, you can simply draw your molecule setting some constraints on the budget, and IBM RXN for Chemistry will explore with its models the chemical space to recommends full synthesis routes.In the interactive mode, IBM RXN for Chemistry acts as an AI-powered assistant: the system recommends disconnections and you choose your favorite option.
Revealing The Effect Of Bias Through Artificial Dataset
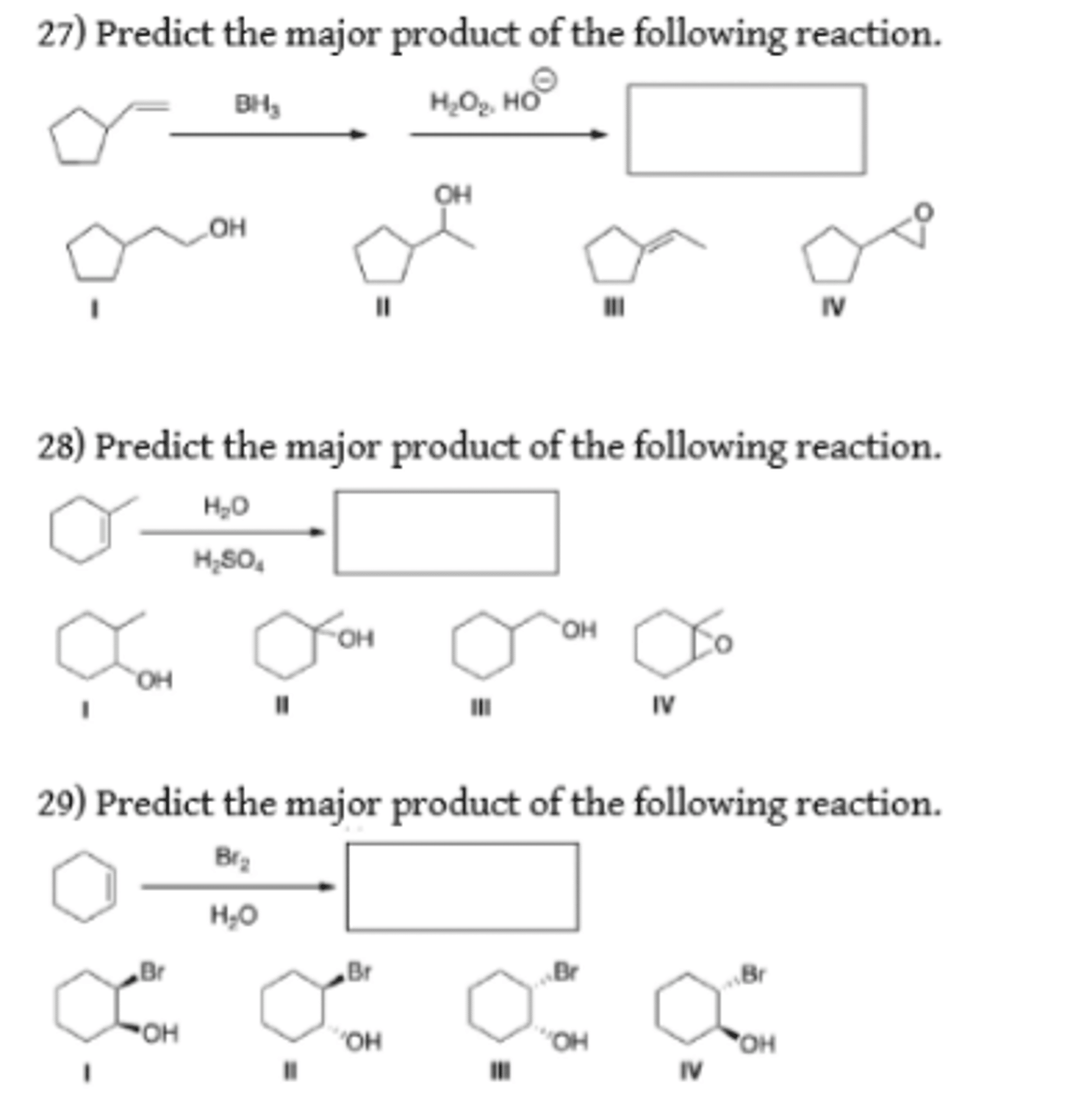
To investigate how imbalance in the training data affects the test set performance, we construct three artificial training sets using reaction templates for meta and para FriedelCrafts substitutions.
The first training set is balanced, containing the same number of para and meta products. The second dataset contains 10% meta and 90% para products, whilst the third dataset has ca 1% meta and 99% para products. This last ratio is closest to the ratios of the USPTO dataset. The test set for all models contains an equal number of meta and para reactions.
Figure b reveals that the Molecular Transformer is highly susceptible to learning dataset bias. When the model is trained on the balanced dataset, it rapidly converges to predicting equal amounts of para and meta substitution reactions, confirming that the bias is not caused by neural network architecture limitations. The model trained on the biased dataset containing only 10% meta reactions in the training set is not able to get rid of the bias fully, but with longer training it is mitigated. For the highly biased training set the model is not able to learn to predict any meta products.
Read Also: What Does Bp Stand For In Biology
Computer System Predicts Products Of Chemical Reactions
*Terms of Use:
Images for download on the MIT News office website are made available to non-commercial entities, press and the general public under a Creative Commons Attribution Non-Commercial No Derivatives license. You may not alter the images provided, other than to crop them to size. A credit line must be used when reproducing images if one is not provided below, credit the images to “MIT.”
Previous imageNext image
When organic chemists identify a useful chemical compound a new drug, for instance its up to chemical engineers to determine how to mass-produce it.
There could be 100 different sequences of reactions that yield the same end product. But some of them use cheaper reagents and lower temperatures than others, and perhaps most importantly, some are much easier to run continuously, with technicians occasionally topping up reagents in different reaction chambers.
Historically, determining the most efficient and cost-effective way to produce a given molecule has been as much art as science. But MIT researchers are trying to put this process on a more secure empirical footing, with a computer system thats trained on thousands of examples of experimental reactions and that learns to predict what a reactions major products will be.
With the new work, Jensen says, the vision is that youll be able to walk up to a system and say, I want to make this molecule. The software will tell you the route you should make it from, and the machine will make it.
Why Is It Important To Predict Products By Determining The Type Of Reaction
Chemical reactions help us understand the properties of matter. By studying the way a sample interacts with other matter, we can learn its chemical properties. These properties can be used to identify an unknown specimen or to predict how different types of matter might react with each other.
Read Also: What Does Relative Frequency Mean In Math
Molecular Orbital Reaction Model
We propose a fundamental reaction unit model starting from the structure of the reactants to enumerate all conceivable primary idealized molecular orbital interactions31 visualizable as arrow-pushing diagrams. This approach yields elementary reaction steps that describe the implied transition state. Other formalisms to describe or enumerate possible chemical reactions exist, such as Dugundji-Ugi,32 Temkin et. al.,33 and Kerber et. al.,34 but these all encapsulate overall transformations, or general graph rearrangements, and none are analogous to such an ubiquitous chemical idea as arrow-pushing.
A molecule m is modeled in the standard manner as a labeled connected molecular graph m = Gm where the vertices Am represent labeled atoms and the edges Bm represent labeled bonds. Then each atom in the graph is augmented with multiple labels to represent approximate electron filled and electron unfilled molecular orbitals . An electron filled MO is defined as the quadruple
fu
The filled and unfilled orbitals yielded for C2. Note the bond adjacent to C4 acts as either a filled or unfilled chain orbital.
Predicting Products Of Chemical Reactions
Predicting Products of Chemical Reactions Worksheet dict the products of the reactions below. Then, write the balanced equation and classify the reaction. If a precipitate forms, indicate by using by the precipitate no reaction occurs write N.R. beside the question. Use your activity series, predicting products helper sheet and the solubility rules. 1. magnesium bromide + chlorine Mg Br + Cl MgCl + Br Al. 2. aluminum + iron oxide 3. silver nitrate + zinc chloride Colo product COO+CO reactant *. cobalt carbonate Coco, 5. zinc + hydrochloric acid 6. sulfuric acid + sodium hydroxide HOFB, INCI + distonic 7. aluminum + oxygen 4 Al +30 + ZALO 8. acetic acid + copper 9. potassium chlorate * KCIO3 + K + CIO, 10. calcium oxide + water CaO + H2O + Ca, 11. nonane + oxygen gas 12. zinc nitrate + potassium iodide
You May Like: What Is Buffer In Biology
Synthesis Process For Predicting Chemical Reactions
The magic behind the app is a set of Language Models based on Transformers that can predict the most likely outcome of a Chemical Reaction or understand natural language description of Chemical Procedures.The service is currently used across several laboratories around the world for automation experiments involving AI, where robots can learn in real-time, based on feedback from IBM RXN and wet-lab experiments. Humans and machines coming together to discover that is powerful.
Close Rankings Are Reasonable
While our ranking method is very accurate, it is not perfect. However, the vast majority of errors are close errors, as exhibited by the 99.89% Within-4 recovery rate. Furthermore, upon examination of these close errors in cross-validation experiments, they are largely intelligible and not unreasonable predictions. For example, Figure 12 shows two reactions involving an oxonium compound and a bromide anion. Across all cross-validation experiments where the reactants are part of the testing set, our predictor ranks these two reactions as the highest, with the deprotonation slightly ahead of the substitution. This is considered a within-1 ranking because from the Reaction Explorer system, only the substitution reaction is labeled productive. However, the immediate precursor reaction in the sequence of Reaction Explorer mechanisms leading to these reactions is the reverse of the deprotonation reaction. Hydrogen transfer reactions like this are reversible, and thus the deprotonation is a reasonable mechanism to predict and rank highly. In this case, the deprotonation is likely the kinetically favored mechanism. It is just not productive, in that it does not lead to the final overall product. In a prediction system attempting to predict multi-step syntheses, such reversals of previous steps are easily discarded.
Also Check: What Difficulty Has Japan Faced Due To Its Geography