Examples Of Correlation Vs Causation
Here are a few quick examples of correlation vs. causation below.
Examples of correlation, NOT causation:
- On days where I go running, I notice more cars on the road.
- I, personally, am not CAUSING more cars to drive outside on the road when I go running. Its just that because I go running outside, I see more cars than when I stay at home. This relationship is not cause-and-effectbecause neither the cars nor I are impacting each other.
Okay, what about an example that may seem more related at first glance:
- On days when I drink coffee, I feel more productive.
- I can feel more productive because of the caffeine, sure. But it can also be because I go to the coffee shop to drink coffee, and I am more productive at the coffee shop than at home when there are a million distractions. This cause-and-effect ISNOT confirmed.
Examples of causation:
- After I exercise, I feel physically exhausted.
- This is cause-and-effect because Im purposefully pushing my body to physical exhaustion when doing exercise. The muscles I used to exercise are exhausted after I exercise . This cause-and-effect IS confirmed.
- When I feed my cat more than 2 treats a day, my cat gets a little chubbier.
- My cat gets fatter because I am feeding it more. This is a cause and effect. Me feeding my cat treats is the cause and the effect is he becomes a little rounder.
Lets pretend that every time I drink coffee, the price of corn in Spain goes up.
But does that magically make it a causal relationship? Nope.
Lewiss Counterfactual Approach To Causation
The philosopher David Lewis has proposed the most elaborately worked out theory of how causality is related to counterfactuals.27 His approach requires the truth of two statements regarding two distinct eventsX and Y. Lewis starts from the presumption that X andY have occurred so that the counterfactual statement,28 If X were to occur, then Y would occur, is true. The truth of this statement is Lewiss first condition for a causal relationship. Then he considers the truth of a second counterfactual:29 IfX were not to occur, then Y would not occur either. If this is true as well, then he says that X causes Y. If, for example, Bismarck decided for war in 1866 and, as some historians argue, German unification followed because of his decision, then we must ask: If Bismarck had not decided for war, would Germany have remained divided? The heart of Lewiss approach is the set of requirements, described below, that he lays down for the truth of this kind of counterfactual.
Causal Relationships Between Variables
What do we mean when we talk about a ârelationshipâ between variables? In psychological research, we’re referring to a connection between two or more factors that we can measure or systematically vary.
One of the most important distinctions to make when discussing the relationship between variables is the meaning of causation.
A causal relationship is when one variable causes a change in another variable. These types of relationships are investigated by experimental research in order to determine if changes in one variable actually result in changes in another variable.
Don’t Miss: What Is Biomass In Biology
Actively Track All The Factors
Track Your Keywords
- Create correlation vs causation worksheet with a list of every single keyword you want to target, next to the page on which youre using these keywords
- Create an excel spreadsheet that records the same information for Top 100 keywords that have generated traffic to your site
Track On-Page Changes
- Every time you make a modification to on-site optimization of any of your webpages, make an annotation in Google analytics
- This annotation should contain details about changes made and the dates on which they occurred
Track the Links
- Create one more Create correlation vs causation worksheet to track the quality and number of inbound links that are reverting to your site
- Make note of the PageRank of the referring URL, root URL, and anchor text used in the link
- Changes in the distribution of such link metrics can cause SEO losses or gains.
Track all social Signals
Lawlike Generalities And The Humean Regularity Approach To Causation
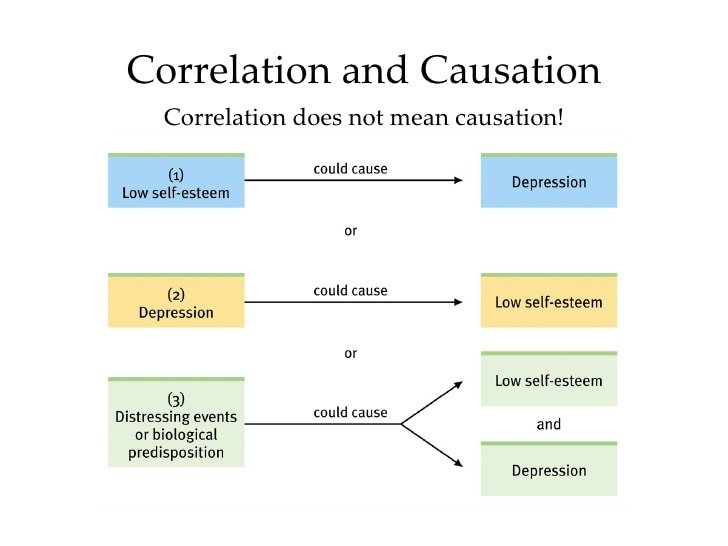
Humean and neo-Humean approaches propose logical conditions that must hold for the constant conjunction of events to justify the inference that they have a causeeffect relationship. Specifically, Humeans have explored whether a cause must be sufficient for its effects, necessary for its effects, or something more complicated.
Fig. 49.1. Two sets of INUS conditions
10.00 |
100.00 |
Read Also: Angle Addition Postulate Homework 4
When Can We Say Causation
If a correlational study doesnt let us choose which causal model is the correct one, there must be some other way, and that other way is called an EXPERIMENT. Unlike a correlational study, which cannot determine which variable comes first, an experiment changes the circumstances to see if the change results in a difference at the end. In scientific terminology, an experiment MANIPULATES one VARIABLE to see if it has an effect on another variable. In other words, is that first variable why the second one changes?
A well-known experiment in psychology tested to see if changing the number of people in a room would influence a person to seek help when smoke starts coming in from under a doorway. In other words, does the number of people who just sit there CAUSE a person to be less likely to seek help for a possible emergency? What we do to test this is bring a participant into a room, ask them to fill out some paperwork, then leave them alone a few minutes later we turn on the smoke machine and smoke comes in from under a side door in the room. We see how long it takes before the person gets up to get help. The experiment is that some participants are seated in the room alone, and some are seated in the room in which five other people are filling out paperwork at other seats, and when the smoke starts, these five other people do nothing special .
Okay, so what do we have there and why is it an experiment that will tell us something about causation?
Randomized And Experimental Study
Say you want to test the new shopping cart in your ecommerce app. Your hypothesis is that there are too many steps before a user can actually check out and pay for their item, and that this difficulty is the friction point that blocks them from buying more often. So youve rebuilt the shopping cart in your app and want to see if this will increase the chances of users buying stuff.
The best way to prove causation is to set up a randomized experiment. This is where you randomly assign people to test the experimental group.
In experimental design, there is a control group and an experimental group, both with identical conditions but with one independent variable being tested. By assigning people randomly to test the experimental group, you avoid experimental bias, where certain outcomes are favored over others.
In our example, you would randomly assign users to test the new shopping cart youve prototyped in your app, while the control group would be assigned to use the current shopping cart.
After the testing period, look at the data and see if the new cart leads to more purchases. If it does, you can claim a true causal relationship: your old cart was hindering users from making a purchase. The results will have the most validity to both internal stakeholders and other people outside your organization whom you choose to share it with, precisely because of the randomization.
You May Like: What Is The Molecular Geometry Of Ccl4
How Do You Test Causality
There is no such thing as a test for causality. You can only observe associations and constructmodels that may or may not be compatible with whatthe data sets show. Remember that correlation is not causation. If you have associations in your data,then there may be causal relationshipsbetween variables.
Relational Or Correlational Research
A study that investigates the connection between two or more variables is considered relational research.The variables that are compared are generally already present in the group or population. For example, a study that looks at the proportion of males and females that would purchase either a classical CD or a jazz CD would be studying the relationship between gender and music preference.
Also Check: What Is Figure Ground Perception Psychology
Correlational Relationships Between Variables
A correlation is the measurement of the relationship between two variables. These variables already occur in the group or population and are not controlled by the experimenter.
- A positive correlation is a direct relationship where, as the amount of one variable increases, the amount of a second variable also increases.
- In a negative correlation, as the amount of one variable goes up, the levels of another variable go down.
- In both types of correlation, there is no evidence or proof that changes in one variable cause changes in the other variable. A correlation simply indicates that there is a relationship between the two variables.
The most important concept is that correlation does not equal causation. Many popular media sources make the mistake of assuming that simply because two variables are related, a causal relationship exists.
Causation Vs Correlation Examples
Lets begin this section with Correlation vs Causation Graph:
As you can see in the graph above, there is a correlation between the amount of ice-cream consumed and the number of people who died because of drowning.
Now, Id sound ridiculous if I say that ice-cream consumption causes drowning, wouldnt I?
In fact ‘Summer Weather is the third variable, which is the causative agent in this scenario.
In warm weather, people tend to consume more ice-cream and also swim more often, which leads to an increase in the number of drowning deaths.
- In this example, Variable X did not cause the manifestation of Variable Y . There was a coincidence. Hence X& Y has a correlation between them.
- If we introduce a Variable Z , then we can see the cause-and-effect relationship between Variable Y and Variable Z . Hence the Z & Y variable has a causation relationship between them.
Also Check: Geometry Seeing Doing Understanding Answer Key
Correlation Is Not Causation
Patterns Of Causality In The Mind
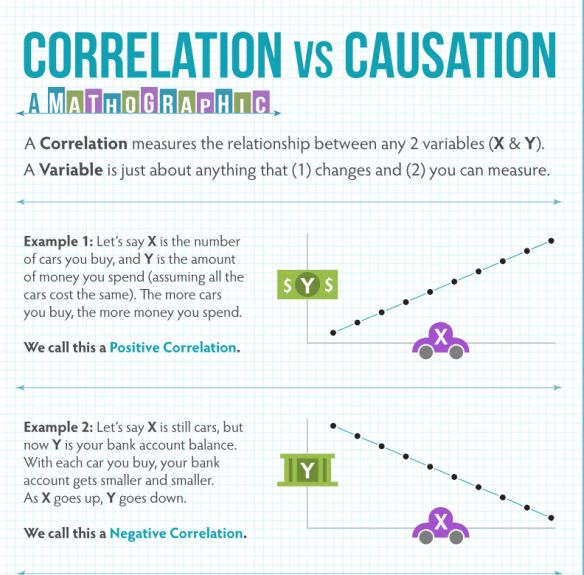
Unfortunately, the human mind is built to try and subconsciously establish links between many contrasting pieces of information. The brain often tries to construct patterns from randomness, so jumps to conclusions, and assumes that a relationship exists.
Overcoming this tendency is part of academic training of students and academics in most fields, from physics to the arts. The ability to evaluate data objectively, is absolutely crucial to academic success.
Read Also: What Does K Stand For In Math
Problems With The Counterfactual Definition38
Although the counterfactual definition of causation leads to substantial insights about causation, it also leads to two significant problems. Using the counterfactual definition as it has been described so far, the direction of causation cannot be established, and two effects of a common cause can be mistaken for cause and effect. Consider, for example, an experiment as described above. In that case, in the treatment group, when C occurs, E occurs, and when E occurs, C occurs. Similarly, in the control group, when C does not occur, then E does not occur, and whenE does not occur, then C does not occur. In fact, there is perfect observational symmetry between cause and effect which means that the counterfactual definition of causation as described so far implies that C causes E and that E causes C. The same problem arises with two effects of a common cause because of the perfect symmetry in the situation. Consider, for example, a rise in the mercury in a barometer and thunderstorms. Each is an effect of high pressure systems, but the counterfactual definition would consider them to be causes of one another.39
Multiple Causes And Mechanisms
Earlier in this chapter, the need to rule out common causes and to determine the direction of causation in the counterfactual approach led us towards a consideration of multiple causes. In this section, the need to solve the problem of pre-emption and the pairing problem led to a consideration of mechanisms. Together, these approaches lead us to consider multiple causes and the mechanisms that tie these causes together. Many different authors have come to a similar conclusion about the need to identify mechanisms , and this approach seems commonplace in epidemiology where debates over smoking and lung cancer or sexual behavior and AIDS have been resolved by the identification of biological mechanisms that link the behaviors with the diseases.
Also Check: What Is The Molecular Geometry Of Ccl4
Here Is A Good Example Of Reverse Causation:
When lifelong smokers are told they have lung cancer or emphysema, many may then quit smoking. This change of behavior after the disease develops can make it seem as if ex-smokers are actually more likely to die of emphysema or lung cancer than current smokers.
It is also known that many alcoholics become nondrinkers after being diagnosed with cirrhosis of the liver. Such changes can confuse or confound what is a causal correlation between dying of a disease and an environmental factor.
Clearly, the decisions by smokers and alcoholics to stop smoking or drinking did not cause them to get sick and die. Even so, this tendency to reduce or eliminate things that make you become sick after the fact can undermine the statistical correlation between smoking and dying of lung cancer, and heavy alcohol consumption and dying of liver disease.
This Article Is A Part Of The Guide:
Causality is the area of statistics that is most commonly misused, and misinterpreted, by non-specialists. Media sources, politicians and lobby groups often leap upon a perceived correlation, and use it to ‘prove’ their own beliefs. They fail to understand that, just because results show a correlation, there is no proof of an underlying causality.
Many people assume that because a poll, or a statistic, contains many numbers, it must be scientific, and therefore correct.
You May Like: Segment Addition Postulate Coloring Activity
The Cost Of Disregarding Correlation And Causation
The principle of incorrectly linking correlation and causation is closely linked to post-hoc reasoning, where incorrect assumptions generate an incorrect link between two effects.
The principle of correlation and causation is very important for anybody working as a scientist or researcher. It is also a useful principle for non-scientists, especially those studying politics, media and marketing. Understanding causality promotes a greater understanding, and honest evaluation of the alleged facts given by pollsters.
Imagine an expensive advertising campaign, based around intense market research, where misunderstanding a correlation could cost a lot of money in advertising, production costs, and damage to the company’s reputation.
Correlation Vs Causation In Mobile Analytics
So what have we learned from all these correlation and causation examples? There are ways to test whether two variables cause one another or are simply correlated to one another.
If youre serious about establishing a causal relationship, then youve got to use the testing method that gives your data and results the most validity. Start with the random experimental design and work your way downwards. And always watch how you think or even verbalize your predictions.
Theres a Latin phrase that goes: Post hoc, ergo propter hoc, which means: After this, therefore because of this. The idea is that by communicating one statement before the other, you imply that the previous caused the latter to happen. The reality is it could just be a correlation or a pure coincidence.
See how todays top brands use CleverTap to drive long-term growth and retention
Read Also: How Does A Hydraulic Lift Work
Psychological And Linguistic Analysis
Although our primary focus is epistemological, our everyday understanding, and even our philosophical understanding, of causality is rooted in the psychology of causal inference. Perhaps the most famous psychological analysis is David Humes investigation of what people mean when they refer to causes and effects. Hume was writing at a time when the pre-eminent theory of causality was the existence of a necessary connectiona kind of hook or forcebetween causes and their effects so that a particular cause must be followed by a specific effect. Hume looked for the feature of causes that guaranteed their effects. He argued that there was no evidence for the necessity of causes because all we could ever find in events was the contiguity, precedence, and regularity of cause and effect. There was no evidence for any kind of hook or force. He described his investigations as follows in his Treatise of Human Nature :
idea
Perspectives On Causal Cognition
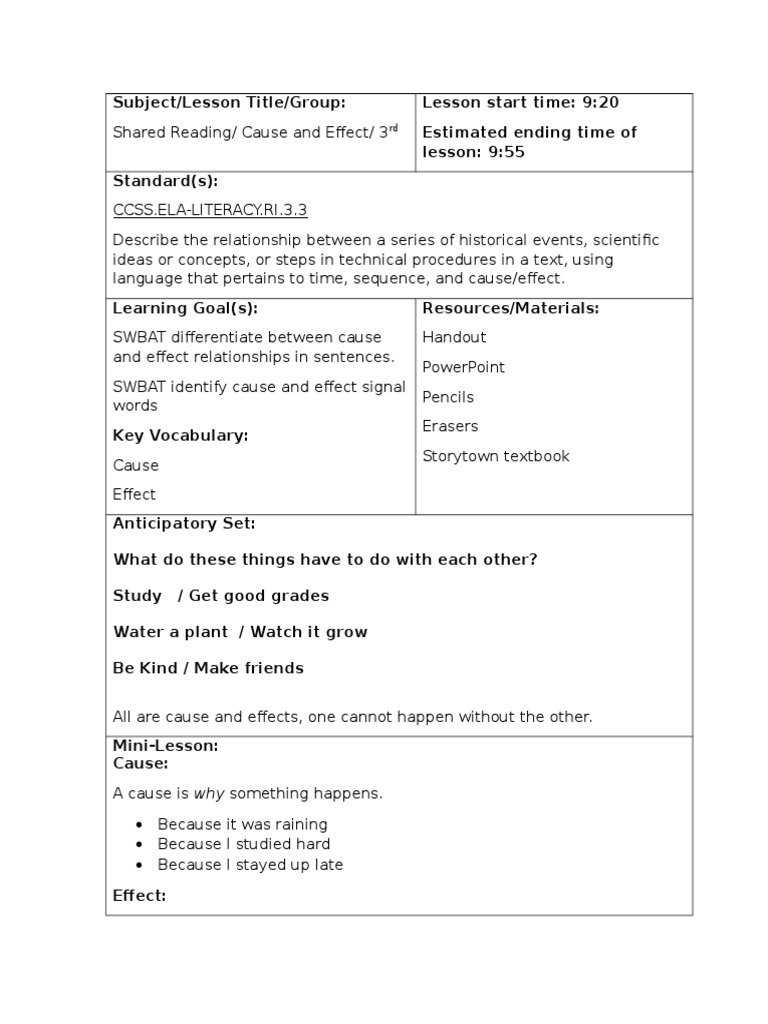
The preamble for this research topic outlines causal cognition as the ability to perceive and reason about cause-effect relations.1 This outline largely reflects what may be seen as the standard view in cognitive and social psychology. In the following, this view will be fleshed out, before addressing the dimensions along which it needs to be extended.
Recommended Reading: Who Are Paris Jackson’s Biological Parents